Autoregressive neural networks for predicting the behavior of viscoelastic materials
DOI:
https://doi.org/10.24352/UB.OVGU-2023-058Keywords:
autoregressive neural network, viscoelasticity, finite element method, deep learning, material model, rubber materialAbstract
In the present work, the capabilities of neural networks to describe viscoelastic material behavior are investigated. Using real one-dimensional test data from a tensile test, autoregressive neural networks were trained. The best networks were then used to calculate the stress and the stiffness in displacement- and force-driven simulations. The results were compared with both experimental data and simulation results of a classical material model.
The viscoelasticity discussed here plays a special role in the description of complex rubber materials, in addition to long-term effects, failure or heat-induced mechanisms. Classical material models simplify the real behavior, which is the reason for the occurrence of simulation errors. To overcome these limitations, this paper presents a different way of material modeling by describing the strain-stress correlation using a neural network. Previous stress states from the time history are used in the calculation to account for the path-dependent behavior of viscoelastic materials. Other effects, such as the influence of different temperatures, are not addressed in this work, but can be included with an appropriately large training data set.
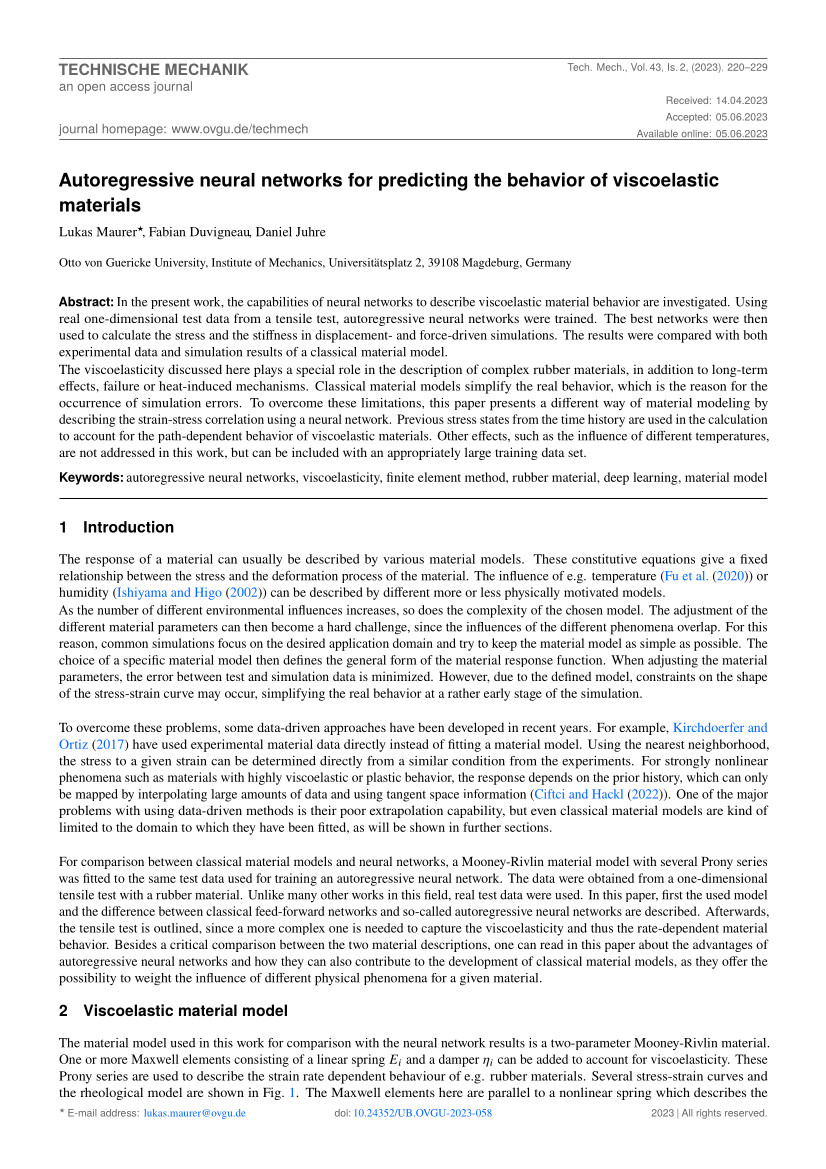
Downloads
Published
How to Cite
Issue
Section
License
Copyright (c) 2023 Lukas Maurer, Fabian Duvigneau, Daniel Juhre

This work is licensed under a Creative Commons Attribution-ShareAlike 4.0 International License.