Convolutional Neural Networks for Time Series Data Processing Applicable to sEMG Controlled Hand Prosthesis
DOI:
https://doi.org/10.24352/UB.OVGU-2024-053Keywords:
Convolutional Neural Network, Feature Engineering, Residual Neural Network, Spatial Feature, Surface Electromyography, Time Series DataAbstract
Surface electromyography (sEMG) signals are often used to control prosthetics, but accurately interpreting these stochastic signals remains challenging. Deep learning tools like convolutional neural networks (CNNs) have shown promise for complex classification problems, yet CNN applications for time series data are limited. This work explores adapting CNNs to sEMG time series for improved classification, addressing two questions: 1) Can a CNN trained on cross-subject data generalize without individualization? 2) Can a small individualized dataset sufficiently train an accurate control model? To investigate, sEMG data is formatted into images using handcrafted features, with pixels representing multichannel time series. A ResNet50 architecture is trained on two datasets: individual and cross-subject. Results show cross-subject models fail to provide accurate subject-specific control due to high inter-subject variability of sEMG. However, ResNet50 trained on individual data produces highly accurate offline and near real-time classification. The proposed method is also tested on an external dataset and compared to similar published methods, demonstrating strong performance. In summary, CNNs show promise for prosthetic control from sEMG but require individualized training data. The proposed data formatting and ResNet50 architecture can enable precise control from minimal data, overcoming barriers to clinical implementation. Further research into cross-subject generalizability is warranted to understand the sources of variability and improve model robustness.
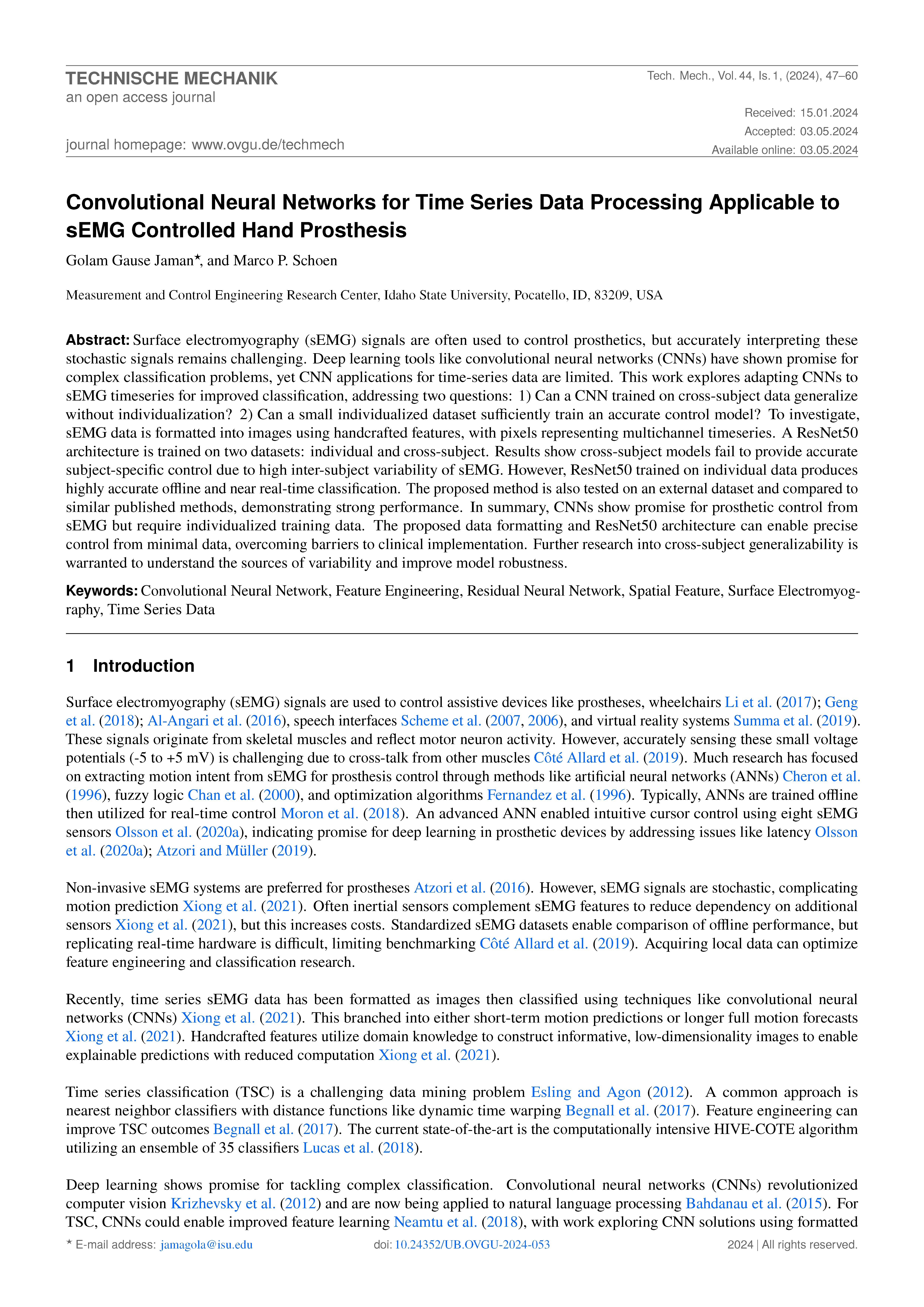
Downloads
Published
How to Cite
Issue
Section
License
Copyright (c) 2024 Golam Gause Jaman, Marco Schoen

This work is licensed under a Creative Commons Attribution-ShareAlike 4.0 International License.